Table of Contents
- 1) Nvidia RTX 3060 (Overall Best)
- 2) NVIDIA Titan RTX (Runner-Up)
- 3) NVIDIA GeForce RTX 3090 (Premium card for Machine Learning)
- 4) Nvidia Tesla K80 (Best machine Learning GPU on Reddit)
- 5) NVIDIA Quadro RTX 5000 (Best Workstation Card)
- 6) Nvidia GeForce RTX 2080 (Price to Performance Card)
- 7) NVIDIA GeForce GTX 1080 (Best budget graphics card)
- Machine Learning FAQs
- Conclusion
“Deep learning GPUs have become quite popular over the recent years mainly because of their utility.”
If you’re starting out your career path in neural networks or machine learning, then the chances are you going to need a powerful setup, preferably one that runs on high-end GPUs. Graphic cards are essential to this whole process mainly because of their massive parallelization, which is quite hard to archive on modern processors.
That’s why today, we will be going through some of the best Nvidia graphics cards for deep learning workstation builds. We will also talk about what can be compromised for your particular use case; so you can get the best bang for your buck. Let’s begin right away!
Here is the list of the Best Deep learning GPUs; · Overall Best graphic card for machine learning: Nvidia RTX 3060 · Runner-Up AI graphics card from Nvidia: NVIDIA Titan RTX · Premium Nvidia GPU for deep learning: NVIDIA GeForce RTX 3090 · Best GPU for machine learning Reddit: Nvidia Tesla K80 · Best Workstation Nvidia GPU for deep learning: NVIDIA Quadro RTX 5000 · Best Price to performance card for machine learning: Nvidia GeForce RTX 2080 · Best budget graphics card for machine learning: NVIDIA GeForce GTX 1080 |
1) Nvidia RTX 3060 (Overall Best)

Framework | Nvidia’s Ampere Architecture |
CUDA Cores | 3584 |
Base Clock | 1.32GHz |
Memory Bandwidth | 1875 MHz |
Interface | 192-bit |
VRAM Config | 12 GB GDDR6 |
Dimensions | Dual-slot |
PCI Express | Gen 4 |
Power Draw | 170W |
Starting out this list at number 1, we have the RTX 3060 from Nvidia. Based on specs alone, this card seems to be the ideal pick for beginners or someone who’s just starting out their machine learning journey. Each unit is paired with plenty of CUDA processors, which will ensure that your datasets load efficiently.
On top of that, the RTX 3060 supports 12GB of DDR4 VRAM, which should be more than enough for any modern tasks (training a StyleGAN). What I personally like about this card is the fact that it’s built under Ampere architecture.
Don’t get me wrong, Nvidia’s Turing framework was also phenomenal, but when you talk about scalability and acceleration, it falls behind Ampere.
This time around, you’ll also get more RT cores, meaning ray tracing is also a possibility if that’s something you fancy. As far as power output goes, it’s relatively stable at 170W, averaging between 70-80 on a greater load.
Because of this, you can build your machine-learning workstation on Nvidia RTX 3060 with ease.
This card is also budget-friendly compared to other GPUs in the 3000 series. You can find deals for it on eBay in the $300-$400 price range. All in all, the RTX 3060 is a great graphic card for machine learning that doesn’t compromise on either performance or affordability.
It has ample CUDA cores, better memory bandwidth, and a VRAM configuration that can set you up for years to come.
PROS | CONS |
Higher CUDA cores for greater parallel computations | Shorter bandwidth than the Ti variant |
Faster AI training with 12GB memory | Not future-proof like the 3090 |
Reasonably priced NVidia GPU for deep learning |
2) NVIDIA Titan RTX (Runner-Up)

Framework | NVIDIA Turing architecture |
Core Count | 4608 CUDA + 576 Tensor Cores |
Base Clock | 1350 MHz |
Memory Bandwidth | 672 GB/s |
Interface | 384-bit |
VRAM Config | 24GB GDDR6 |
Dimensions | Dual-slot |
PCI Express | Gen3 |
Power Draw | 280 Watts |
When it comes to machine learning, your hardware will mostly gravitate toward gamer-oriented. However, that’s not the case with the next card on our list which is non-other than the Nvidia Titan RTX.
Nvidia’s separate line of GPUs is aimed at data scientists or anyone who wants to train AI over larger datasets.
The great thing about this piece of hardware is the fact that it comes with both CUDA cores and Tensor processors. This means you’ll have added performance in parallel workloads.
Besides that, each card is packed with an astronomical 24GB of VRAM, which helps you to dedicate extra juice to run tasks simultaneously.
It’s also not bulky and can be acquired in a 2-slot space. As for the cooling, it uses its twin-fan technology to keep the temperatures moderate. Another thing to keep in mind is that this GPU can drain a lot of power, so keeping a PSU of 650W is recommended.
The other I dislike about this ai graphics card is its hefty price tag. Even at MSRP, it is tagged at $2500, which is quite a lot.
In conclusion, the NVIDIA Titan RTX is a beast of a GPU that can handle video encoding, machine learning, and research analysis on the go. As long as you have the budget, I will recommend this card in your next deep learning build.
PROS | CONS |
It runs under the NVIDIA Turing architecture. | It’s clearly an expensive card |
Greater number of CUDA cores for machine learning | Poor cooling/ventilation |
Ultra-fast 24GB of VRAM |
3) NVIDIA GeForce RTX 3090 (Premium card for Machine Learning)
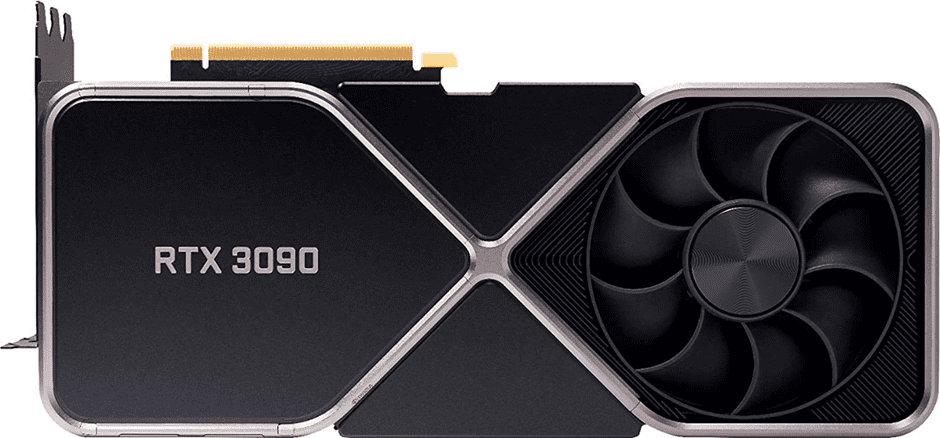
Framework | Nvidia’s Ampere Architecture |
CUDA Cores | 10496 |
Base Clock | 1.40GHz |
Memory Bandwidth | 1008.3 GB/sec |
Interface | 384-bit |
VRAM Config | 24GB GDDR6X |
Dimensions | 3-Slot card |
PCI Express | Gen 4 support |
Power Draw | 350 |
If performance is something you fancy in the premium GPU lineup, then there is no card that come as close to Nvidia RTX 3090. It’s a powerful piece of hardware that delivers up to the standards especially when budget is not a concern.
It has more than triple the CUDA cores compared to RTX 3060 as well as support for 3rd gen Tensor processors.
This will ensure you have the raw compute power no matter what ML task you throw at it. On the surface, it’s all metal, looks incredible, and weighs almost 5 pounds. Keep in mind that it’s a 3-slot GPU, so that you will need extra space in your deep learning workstation.
Nonetheless, it can crunch and process so much data and that’s thanks to its 24GB onboard memory.
Of course, you won’t be needing that much VRAM for gaming but for ML workloads (like object detection), this might come in handy. Another cool thing that you will get with every 3000 series Nvidia GPU is support for parallel computing.
Many of today’s applications take advantage of CUDA cores for machine learning so having an RTX 3090 is definitely a plus.
With that said, there are a couple of shortcomings in the 3090 Nvidia GPU for deep learning. First, you have to take into account how much heat this thing generates.
It has a TDP of 350W, so that you will need a 700W power supply. Secondly, there is also the availability factor that comes with every 3090 GPU, at least for now.
Apart from that, the RTX 3090 demolishes every benchmark, whether machine learning, data analysis, 4K video rendering, or gaming.
PROS | CONS |
Top-of-the-line graphic card for machine learning | It requires quite a lot of power |
Substantial memory bandwidth | Hard to find it on MSRP |
Suited for professionals |
4) Nvidia Tesla K80 (Best machine Learning GPU on Reddit)

Framework | Kepler Architecture |
CUDA Cores | Approx 5000 CUDA cores |
Base Clock | 810 MHz |
Memory Bandwidth | 480 GB/s |
Interface | 384-Bit |
VRAM Config | 24GB GDDR5 |
Dimensions | Dual-slot card |
PCI Express | 3.0 x16 interface |
Power Draw | 300W |
The Nvidia Tesla K80 was introduced in November of 2014 as a data center compute GPU. It’s a dual-GPU card featuring a pair of GK-210-based second-generation Kepler cores.
Each unit also supports 2496 CUDA cores (dual channel) with a base clock of 562 MHz and a boost of 824GHz. On top of that, it has 24GB of GDDR5 memory clocked at 2500MHz.
The K80 has support for all modern graphics APIs such as Direct X12, Vulcan, and OpenGL 4.6. This means that despite being 7-8 years old, it should still be capable of running modern applications.
The dual-channel VRAM also helps it load deep learning models for object detection, object segmentation, or pause segmentation.
Compared to most Nvidia GPUs for deep learning, the K80 is also cost-effective. A brand-new model can be brought for around $350, which is quite a bargain. There are no graphical outputs on Nvidia Tesla K80 and that’s for a specific reason.
It’s intended to be used only as a compute GPU so things like plugging away scientific applications, genome breakdowns, object detection, or financial data can be archived with greater efficiency.
As far as issues are concerned, the Nvidia Tesla K80 supports blower-style fans. This means you don’t want to be sitting in the same room as this card, even with a good set of noise cancellation headphones on.
Similarly, you can’t hook up a monitor directly on this card, and playing games on this GPU will require some trial and error. Nevertheless, for machine learners, this card might just be worth the headache.
PROS | CONS |
Supports CUDA for parallel processing | Setting up the K80 is quite the hustle. |
Made specifically for data analysis and AI training | Quite difficult to game on |
Great value for money |
5) NVIDIA Quadro RTX 5000 (Best Workstation Card)
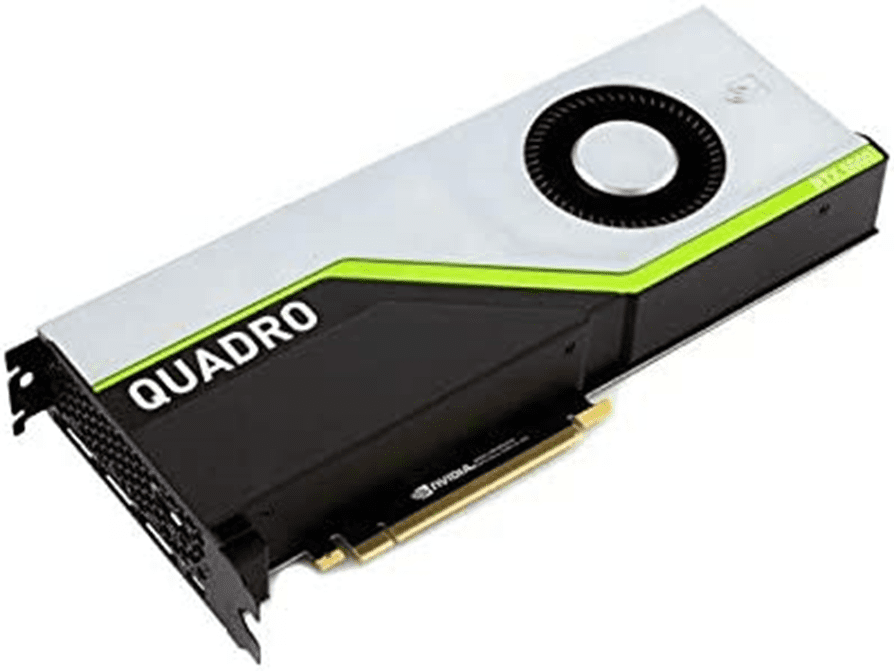
Framework | NVIDIA Turing™ architecture |
Core Count | 3072 CUDA + 384 Tensor Cores |
Base Clock | 1620 MHz |
Memory Bandwidth | 448 GB/s |
Interface | 256-Bit |
VRAM Config | 16GB GDDR6 |
Dimensions | Dual-slot card |
PCI Express | Gen 3 |
Power Draw | 230 W |
Next on the list we have the Quadro RTX 5000; a workstation GPU targeted toward large content creation studios, data scientists as well as deep learning. If you want something to pair in your multi-GPU machine learning build, then the RTX 5000 is worth the purchase.
On the surface, this might be an enterprise-level card based on Nvidia’s Turing architecture, but on closer inspection, you will find it quite special.
With 3072 CUDA cores and 384 Tensor processors, this card packs serious performance. No matter what your workloads are, you won’t be limited, especially in the graphics department. It even has room for 16GB on-board memory, which is excellent for handling larger data sets.
Another great thing about this GPU is its availability. As you know, buying a graphic card in 2022 is a nightmare, but that isn’t the case with RTX 5000 for several reasons. For once, the 230W TDP on this card makes mining a nightmare, and it isn’t good for gaming as well because of drivers and output issues.
Similarly, it has support for NVLink technology which enables it to be connected with multiple cards in a machine. This will further upgrade your performance for complicated projects.
In terms of price, it’s still on the higher end, coming at around $1900 on Amazon. But if you want serious rendering power in professional workloads, then this is the card you should look out for.
PROS | CONS |
Real-time acceleration | At around $1900, it isn’t cheap |
Provided with NVLink technology | No HDMI output |
Supports Ray tracing |
6) Nvidia GeForce RTX 2080 (Price to Performance Card)

Framework | Nvidia Turing Architecture |
CUDA Cores | 2944 CUDA cores |
Base Clock | 1515MHz |
Memory Bandwidth | 448 GB/s |
Interface | 256-bit |
VRAM Config | 8GB GDDR6 |
Dimensions | 2-Slot card |
PCI Express | Gen4 |
Power Draw | 215W |
Not everyone has the budget to spend over $1000 on deep learning GPUs and if your one of them then Nvidia’s RTX 2080 lineup is your best bet.
Based on Turing architecture, this card is great for training neural networks or loading small-scale development projects. It comes with just a shy of 3000 CUDA cores which will help you out in parallel processing.
In addition to that, the RTX 2080 requires only 215W to operate, so it’s not power hungry by any means. As for performance, it even shares a handful of RT cores for those who want to test out their ray-tracing technology. Another great thing about this GPU is that it’s made for multi-purpose applications.
You will see it having HDMI support along with a plethora of IO that you are going to be needing in your small-scale ML setup. Speaking of setup, like many cards in the 2000 series, this one is available in a 2-slot configuration. It’s not enormous, so even users with a compact casing will be able to benefit.
Overall, if you’re looking for that price to performance sweet spot, then the RTX 2080 is a great investment. The only thing you need to be concerned about is its graphics memory which isn’t close to something like the Tesla K80. But that aside, it’s truly one of the best graphic cards for machine learning.
PROS | CONS |
Packs a reasonable compute power | 8GB memory is not recommended for machine learning |
Made for power efficiency | With a little bit of investment, you could get the RTX 3060 |
Its DLSS compatible |
7) NVIDIA GeForce GTX 1080 (Best budget graphics card)
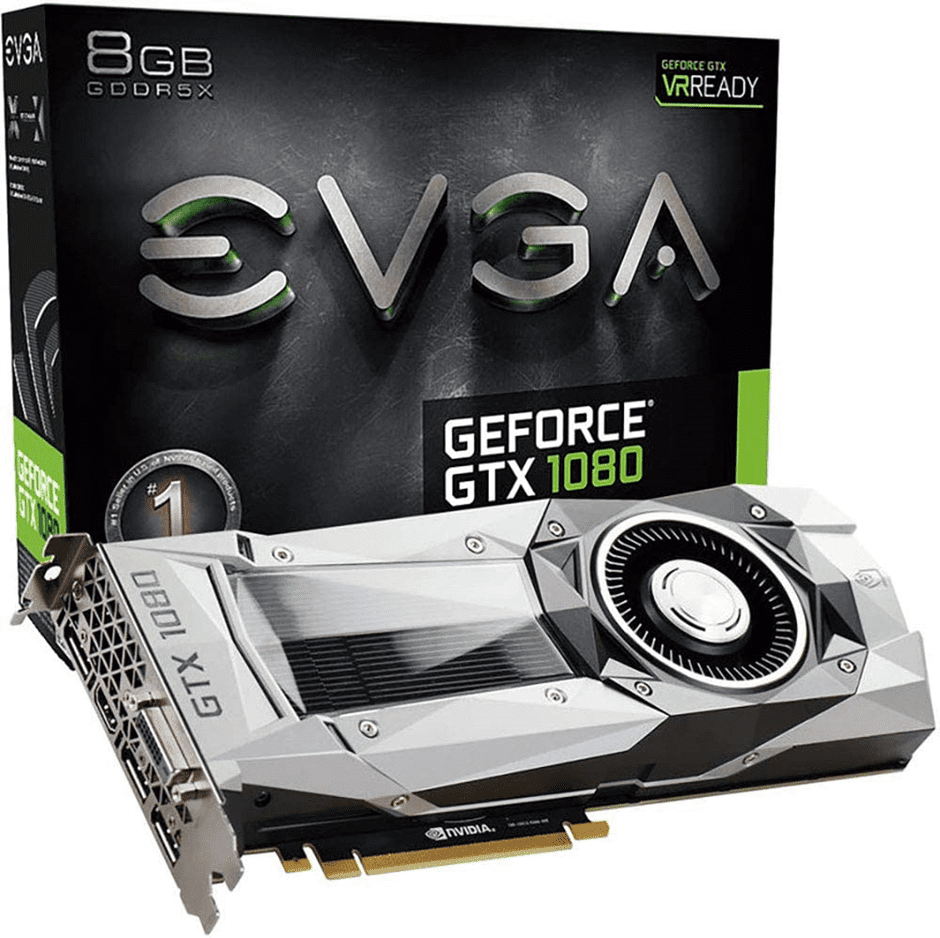
Framework | Nvidia Pascal Architecture |
CUDA Cores | 2560 CUDA |
Base Clock | 1607 |
Memory Bandwidth | 320 GB/sec |
Interface | 256-bit |
VRAM Config | 8GB GDDR5X |
Dimensions | 2-Slot card |
PCI Express | PCIe 3.0 |
Power Draw | 180 W |
Now deep learning computers can be cheap as well and the GTX 1080 from Nvidia clearly proves that. Even in 2022, its an affordable GPU that should be able to train your AI models. Like its predecessors, its CUDA enabled meaning you will be able to load programs like PyTorch with greater efficiency.
On top of that, its architecture named Turing uses a technology called Tensor cores to accelerate deep learning inference.
As for the cooling, it uses a single blower style fan which, in theory, will be able to limit thermal throttling. So, if you want to use this card with an SLI bridge, there is an opportunity.
One thing to note about the NVIDIA GTX 1080 is that it only got 8GB of internal RAM. What that means is that it’s going to be easier to run into memory issues if your aim is to train large models on big datasets.
But as long as you stay with smaller batches, you should be able to avoid this tradeoff. Furthermore, if you like 1080p gaming on medium settings, it still supports that. Just don’t expect miracles out of this Pascal architecture, and other than that, you are good to go.
So, for those looking to build a brand-new deep learning setup under just $1000, the GTX 1080 is worth your money. It has everything built right into it at a cost that won’t limit your choice.
PROS | CONS |
An affordable Nvidia GPU for deep learning | Its 8GB VRAM will be limited in many ML projects |
256-Bit memory interface | Pascal architecture is becoming irrelevant. |
Works well with CUDA library |
Machine Learning FAQs
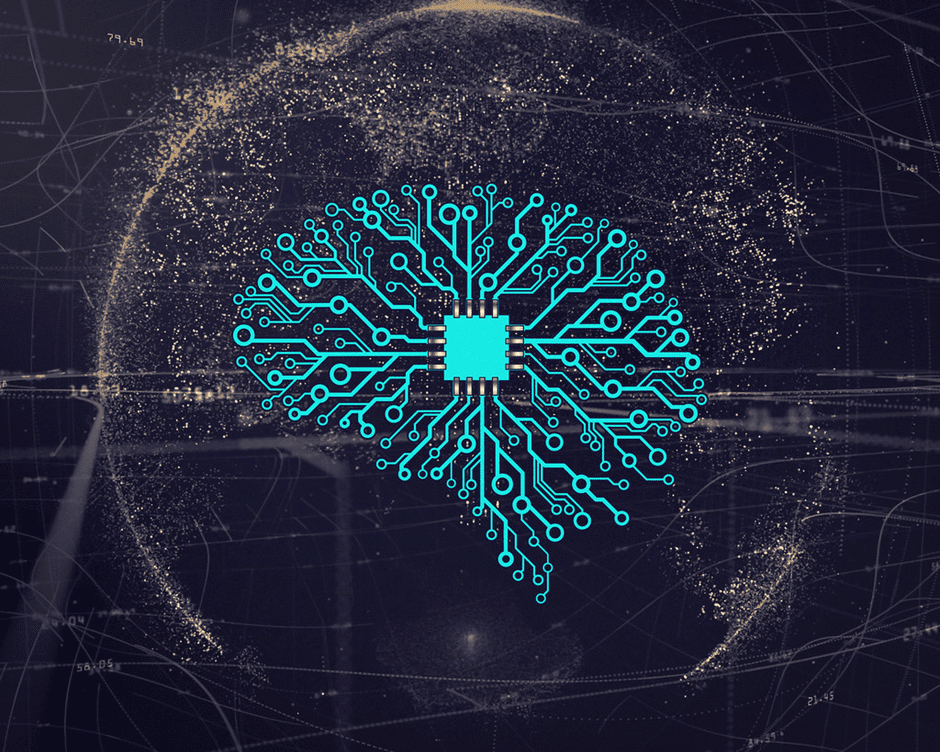
Q1; Do I need a graphic card for machine learning; if so, then why?
Yes, you definitely do. Essentially the way in which they are built makes them perfect candidates to do a lot of efficient matrix operations which end up important in deep learning.
They are ideal for this particular use case over something like a CPU because they can transfer large amount of information from memory at a particular time.
Comparing this to a CPU, you will find them relatively fast but they can’t transfer as much. So, instead of having four, six or eight parallel processes graphics card are able to have hundreds if not thousands of parallel processes, each transferring large batches of data from memory.
Also, Nvidia chips are going to have several thousands of CUDA cores on a single graphics card. Compared that to between 4 and 12 processors for a certain CPU.
So, to recap, graphics cards have vastly more cores. They might be a bit slower for a particular cycle, but they have a much larger bandwidth, so the information that can be carried with each process is going to be higher than a CPU. This is why graphics cards are a necessity in machine learning.
Q2, Why opt for Nvidia GPUs for deep learning?
You might have realized that I haven’t recommended any AMD graphics card in this list. The reason is not that I am biased toward Nvidia but because of their proprietary technologies like CUDA. It is basically a framework that acts as a bridge between Nvidia GPUs and GPU-based applications.
This allows TensorFlow and PyTorch (two of the most popular deep learning libraries) to be able to keep you accelerated, which is exactly the reason why you should be using a GPU for deep learning in the first place.
Q3, How many GPUs are needed for machine learning?
Ideally, you should be looking for two or more GPUs if your aim is to build a machine learning rig. These graphics cards linked in SLI will ensure you get enough computation to train your AI for a variety of projects, including object detection, small-scale weather prediction, drug analysis, and much more. You could also go for virtual computation like Google Cloud if budget is a big constraint.
Also take a look at Computer specs for Python programming.
Things to Consider While Buying Deep Learning GPUs
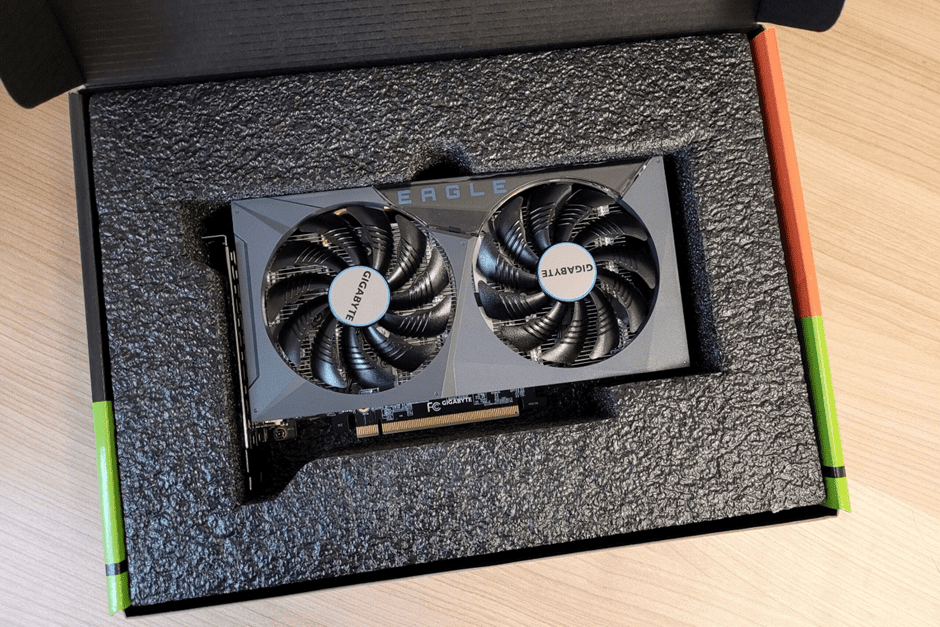
VRAM
- Having a huge amount of dedicated RAM can go a long way especially if your aim is to train AI (such as the case in StyleGAN). A lot of graphics cards these days are going to have several gigabytes of dedicated RAM. The cards that are recommended above will have 10GB or more of dedicated memory and that’s going to be sufficient for a lot of model training.
Cores
- The number of CUDA cores in your GPU will determine how many parallel computations you can perform per batch. As a rule of thumb, the higher the better but anything above than 3000 CUDA range is what I would recommend.
Cooling
- In a multi-GPU setup, its important to look for a proper cooling mechanism as that will help you against thermal throttling. Any ai graphics card with a blower-style fan will be efficient in keeping core temperatures low without affecting your overall performance.
Conclusion
This article was basically aimed at any average person looking to get into deep learning. Before you leave, let me summarize it again. So, the main considerations for the best graphic card for machine learning are going to be dedicated ram and CUDA cores. Taking into account both of these factors, the best card for the price is going to be the most in-demand, and that is the RTX 3060.
These are outstanding machine learning cards, and they will definitely deliver in the long run. If you want something more exclusive, then my recommendation will be the Titan RTX. These professional grade cards are ideal for someone who has trained neural networks before and knows they are going to be heavily involved in the space.